Machine Learning Solutions for Predictive Analytics: A Game-Changer for Decision-Making
Businesses are inundated with vast amounts of information. The challenge lies not just in collecting this data, but in extracting actionable insights from it. Enter machine learning solutions, a transformative technology that enables organizations to harness data for predictive analytics. By leveraging these advanced techniques, businesses can anticipate future trends, optimize operations, and make informed decisions. In this article, we will explore how machine learning solutions are revolutionizing predictive analytics, the role of AI development companies, and the significance of enterprise AI development in shaping strategic decisions.
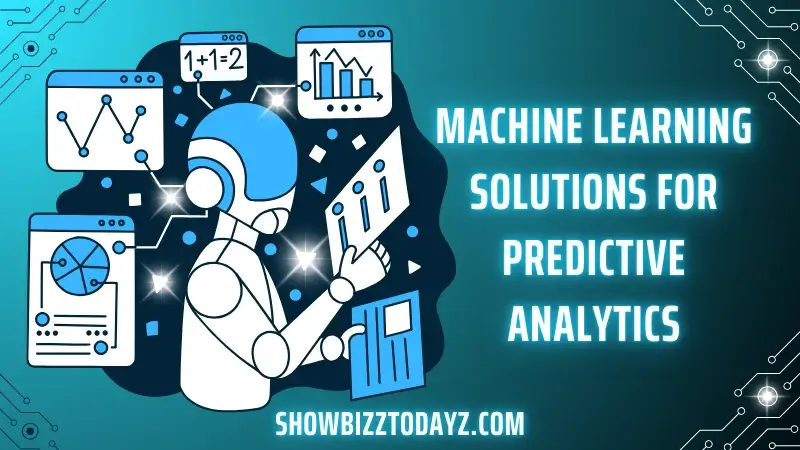
Understanding Predictive Analytics
Predictive analytics refers to the practice of using statistical algorithms and machine learning techniques to identify the likelihood of future outcomes based on historical data. By analyzing patterns and trends, organizations can forecast events, customer behavior, and market dynamics. This approach is pivotal in various sectors, including finance, healthcare, retail, and marketing. Visit the website to know more.
The Importance of Predictive Analytics
- Informed Decision-Making: Predictive analytics empowers businesses to make decisions based on data rather than intuition. This leads to more accurate forecasts and reduced risk.
- Cost Efficiency: By anticipating trends and customer needs, organizations can optimize resource allocation, reducing waste and improving operational efficiency.
- Enhanced Customer Experience: Understanding customer behavior enables businesses to tailor their offerings and improve service delivery, leading to heightened customer satisfaction and loyalty. Read another trending article, 5 Things That Make No Sense in The Naruto Franchise.
Key Components of Predictive Analytics
- Data Collection: Gathering relevant data from various sources, including internal databases, customer interactions, and external market research.
- Data Preparation: Cleaning and organizing data to ensure quality and reliability for analysis.
- Modeling: Applying statistical algorithms and machine learning techniques to build predictive models.
- Evaluation: Assessing model performance to ensure accuracy and reliability in predictions.
- Deployment: Implementing the predictive model in real-world scenarios to generate insights and guide decision-making.
How Machine Learning Solutions Enhance Predictive Analytics
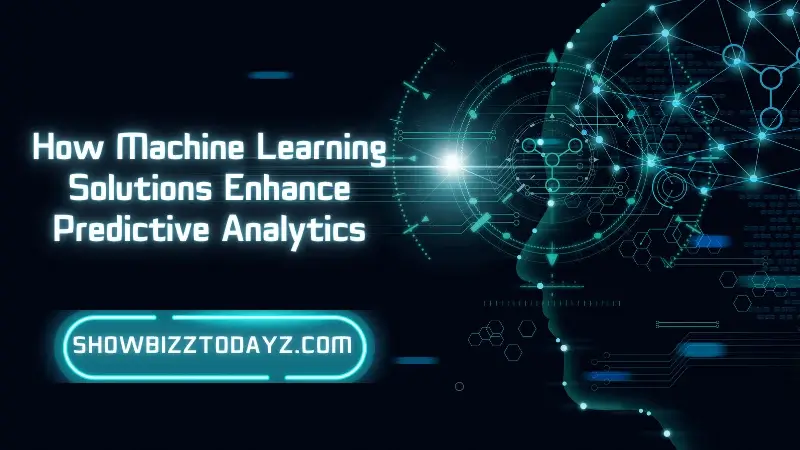
Machine learning solutions play a crucial role in enhancing predictive analytics by automating data analysis and improving accuracy. Here are some ways they contribute:
1. Improved Accuracy
Machine learning algorithms can analyze vast datasets more efficiently than traditional methods. They can identify complex patterns and relationships in the data that might be overlooked by human analysts. This leads to more accurate predictions, allowing businesses to make better-informed decisions.
2. Real-Time Insights
With the ability to process data in real-time, machine learning solutions enable organizations to respond quickly to changing market conditions. This agility is essential in competitive industries where timing can significantly impact success. Check out our comprehensive article on Evolution of E-Bikes for additional information.
3. Scalability
As organizations grow, so does their data. Machine learning solutions can scale to accommodate increasing volumes of data without a corresponding increase in manual analysis efforts. This scalability ensures that predictive analytics remains effective as businesses evolve.
4. Automation of Processes
Machine learning solutions automate repetitive tasks such as data cleaning and model training, freeing up analysts to focus on strategic initiatives. This not only enhances efficiency but also allows organizations to deploy predictive analytics more broadly across their operations.
Applications of Machine Learning Solutions in Predictive Analytics
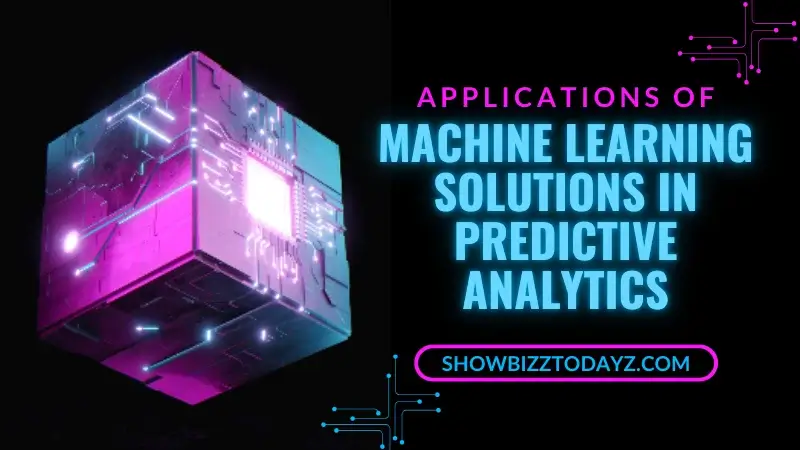
1. Financial Services
In the financial sector, machine learning solutions are instrumental in credit scoring, fraud detection, and risk assessment. By analyzing transaction patterns and customer behaviors, financial institutions can make informed lending decisions and identify potential fraud in real-time.
2. Healthcare
Predictive analytics in healthcare can lead to improved patient outcomes. Machine learning algorithms can analyze patient data to predict disease outbreaks, readmission risks, and treatment effectiveness, enabling healthcare providers to deliver proactive care.
3. Retail
In retail, machine learning solutions enhance inventory management, sales forecasting, and customer segmentation. By predicting buying patterns, retailers can optimize stock levels, tailor marketing campaigns, and improve the overall shopping experience. Enhance your understanding by reading our in-depth post on Aftermarket Solutions for EGR Delete.
4. Marketing
Predictive analytics in marketing aids in customer targeting and campaign optimization. Machine learning algorithms can analyze customer data to predict which segments are most likely to convert, allowing marketers to allocate resources effectively and drive higher ROI.
The Role of AI Development Companies
AI development companies play a pivotal role in helping organizations implement machine learning solutions for predictive analytics. These companies provide expertise in developing algorithms, data management, and system integration tailored to specific business needs.
How AI Development Companies Contribute
- Custom Solutions: AI development companies can create bespoke machine learning models tailored to the unique requirements of a business, ensuring optimal performance and relevance.
- Expertise and Support: These companies bring specialized knowledge in data science, machine learning, and industry best practices, guiding organizations through the implementation process.
- Access to Advanced Technologies: AI development companies often leverage cutting-edge tools and technologies that may not be readily available to in-house teams, enhancing the overall effectiveness of predictive analytics initiatives.
Choosing the Right AI Development Company
When selecting an AI development company, organizations should consider:
- Experience and Expertise: Look for companies with a proven track record in machine learning and predictive analytics.
- Industry Knowledge: Choose a partner that understands the specific challenges and opportunities within your industry.
- Scalability: Ensure the company can provide solutions that can grow with your business.
The Importance of Enterprise AI Development
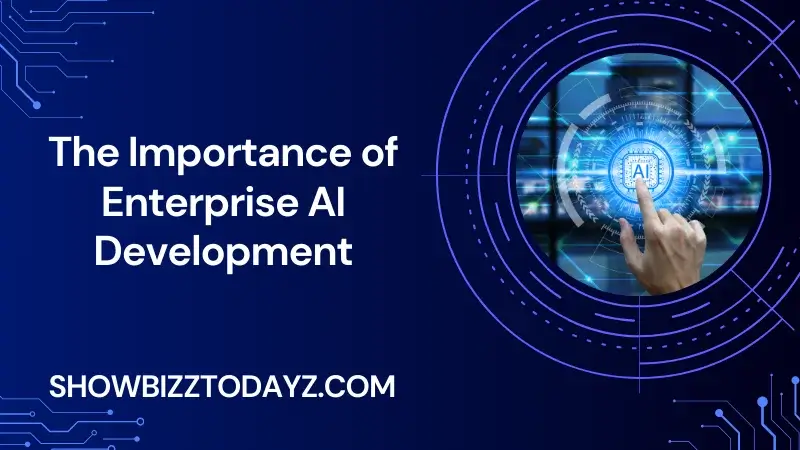
For larger organizations, enterprise AI development company services are crucial for implementing machine learning solutions effectively. This involves creating scalable, secure, and integrated AI systems that can handle complex data environments.
Key Considerations in Enterprise AI Development
- Integration with Existing Systems: Enterprise AI solutions must seamlessly integrate with a company’s existing IT infrastructure to ensure smooth operations and data flow.
- Data Governance: Establishing robust data governance practices is essential for maintaining data quality, security, and compliance with regulations.
- Scalability and Flexibility: Enterprise AI solutions should be designed to scale as the organization grows, accommodating increasing data volumes and user demands.
- User Training and Adoption: Ensuring that employees are trained to use AI-driven tools effectively is crucial for maximizing the benefits of predictive analytics.
Challenges in Implementing Machine Learning Solutions
While machine learning solutions offer significant advantages for predictive analytics, organizations may face several challenges during implementation:
1. Data Quality and Availability
The effectiveness of predictive analytics hinges on high-quality data. Organizations must ensure they have access to relevant, accurate, and timely data to train their machine learning models.
2. Complexity of Models
Machine learning algorithms can be complex, requiring specialized knowledge to develop and interpret. Organizations must invest in training or hire skilled data scientists to manage these models effectively.
3. Resistance to Change
Cultural resistance to adopting new technologies can hinder the implementation of machine learning solutions. Organizations need to foster a data-driven culture to encourage acceptance and utilization of predictive analytics.
4. Ethical Considerations
The use of machine learning in predictive analytics raises ethical questions, particularly concerning data privacy and bias. Organizations must ensure their algorithms are fair and transparent, mitigating potential risks.
Future Trends in Predictive Analytics and Machine Learning
As technology continues to evolve, several trends are shaping the future of predictive analytics and machine learning solutions:
1. Increased Automation
The automation of machine learning processes will continue to grow, allowing organizations to deploy predictive analytics more efficiently and at scale. Automated machine learning (AutoML) tools will simplify model development and deployment.
2. Enhanced Interpretability
As businesses demand more transparency in AI-driven decisions, the focus on interpretable machine learning will increase. Solutions that provide insights into how models make predictions will build trust and facilitate better decision-making.
3. Integration with IoT
The integration of predictive analytics with IoT devices will enable real-time insights and decision-making across various industries. This combination will facilitate proactive responses to changing conditions and optimize operations.
4. Personalization
Predictive analytics will increasingly drive personalization in customer experiences. Businesses will leverage machine learning to tailor offerings and communications based on individual preferences and behaviors.
Conclusion
Machine learning solutions for predictive analytics are indeed game-changers for decision-making across industries. By harnessing data-driven insights, organizations can make informed decisions, optimize operations, and improve customer experiences. The collaboration with AI development companies and the emphasis on enterprise AI development further enhance the efficacy of these solutions.
As organizations embrace machine learning, they must navigate challenges related to data quality, complexity, and ethical considerations. The future of predictive analytics is bright, marked by trends such as increased automation, enhanced interpretability, and integration with IoT technologies. By leveraging these advancements, businesses can position themselves for success in an increasingly competitive landscape.
In conclusion, the transformative power of machine learning solutions in predictive analytics cannot be overstated. By investing in these technologies and collaborating with the right partners, organizations can unlock their full potential, driving innovation and growth in the digital age. You can visit showbizztoday.com for more trending posts.